This project has now finished. It was led by Dr Tingting Zhu at the University of Oxford.
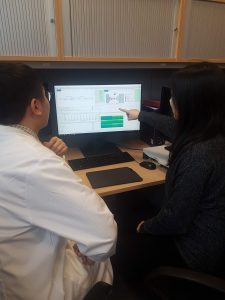
PI Dr Zhu (right) explaining the automated decision support tool for ECG analysis to Dr Jin Liu (left) from Guangzhou General Hospital (GGH) during his visit to Oxford.
Cardiovascular diseases (CVD) cause 17.7 million of deaths annually across the globe, according to the World Health Organisation. CVD is especially prevalent in China, accounting for 45% of all deaths annually, with myocardial infarction (MI) as the most common cause. CVD also has an associated financial burden of $558 bn. The common diagnosis of MI requires clinicians to provide inspection of electrocardiogram (ECG) waveforms and blood tests. Diagnosis is difficult to achieve in a timely manner due to the slow generation of results from laboratory tests, as well as the inter-observer variability in ECG interpretation, resulting in disagreement of diagnosis. The ECG can be noisy or artefactual; its interpretation is highly dependent on the expertise and training of the clinician. The scarcity of expertise results in inequities in access to clinicians with appropriate training, delayed treatment, and increased risk of mortality. There is a need for an automated system that can reduce inter- and intra-expert variability in MI diagnosis, and where it would be required to improve care in resource-constrained settings. This project produced proof-of-principle machine learning algorithms, utilising only ECGs without the need of blood tests, that serve as an automated decision support tool, for diagnosing patients at risk of MI as well as predicting the on-set of MI.
This work and its findings are presented in a video by the Project Investigator here: